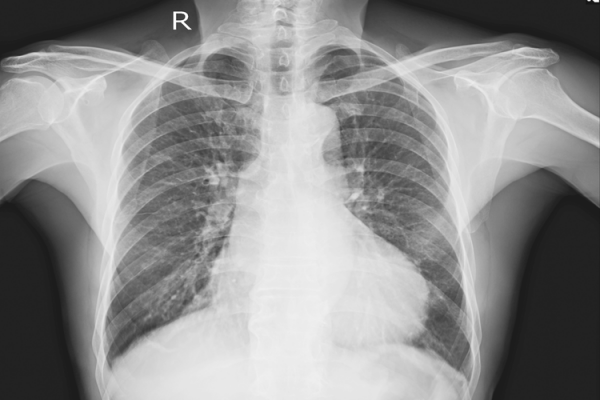
A team from Michigan Medicine and the Michigan Center for Integrative Research in Critical Care (MCIRCC) is using a new artificial intelligence algorithm to analyze chest x-rays for Acute Respiratory Distress Syndrome (ARDS), a life-threatening lung injury that progresses rapidly and can often lead to long-term health problems or death.
“In our previous work, we found that physicians have difficulty identifying findings of ARDS on chest x-rays,” says Michael Sjoding, M.D., a pulmonary critical physician at Michigan Medicine and lead author of the study. “Early recognition and treatment are key factors in treating ARDS. Delays can be catastrophic.”
In an approach called transfer learning, the research team first trained a type of machine-learning model call deep convolutional neural networks, or CNNs, with 121 layers and 7 million parameters, to identify common radiologic findings, but not ARDS, on 450,000 chest x-rays from publicly available sources. Then they trained the algorithm to detect ARDS using a unique dataset of 8,000 chest x-ray studies carefully reviewed and annotated for ARDS by Michigan Medicine physicians. In the study, published in Lancet Digital Health, the algorithm showed that it could, in fact, identify ARDS findings with higher accuracy than many physicians, and it also performed well when it was externally validated in patients from another hospital system.
The team envisions this technology will help physicians identify ARDS patients more quickly and accurately, and ensure patients receive evidence-based care. The tool could also accelerate ARDS research, Sjoding notes, “We now have a highly reliable way to identify ARDS patients, which will also allow us to study them more effectively.”
Read the full article at: Deep Learning Algorithm Detects Acute Respiratory Distress Syndrome with Expert-Level Accuracy (uofmhealth.org)