Abigail is an intern with ITS Marketing and Communications. One of her projects was to create and test a Maizey personalized to a topic of interest. She chose to create one to analyze ITS social media engagement. This is the first article in a series detailing the development and application of this chatbot.
With social media becoming the battlefield for brand attention and engagement, understanding the factors of online interactions is not just helpful; it’s essential. As a marketing intern for U-M’s ITS department, I’ve seen first-hand how social media’s ever-changing landscape requires intricate analysis to harness its full influence. Given ITS’s recent dedication to social media as an information and communication tool and the tech-savviness of our department, I recognized an opportunity to train a Maizey, a U-M AI chatbot trained on your own data, to intricately analyze our social media metrics.
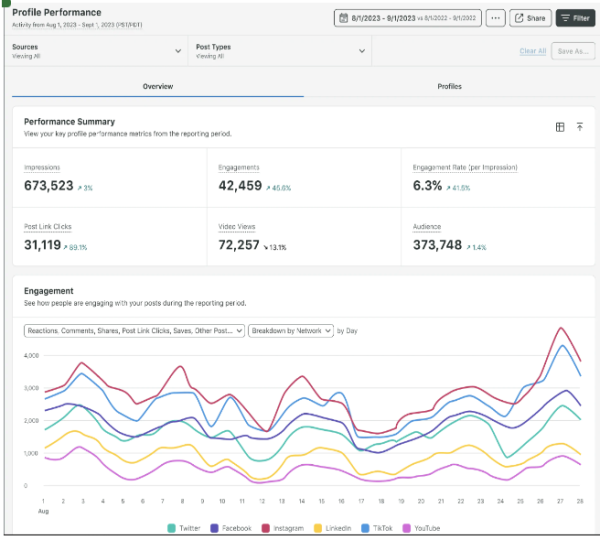
To gain insight into our current strategies, I collaborated with Tommy Valdez, the social media specialist for ITS. Tommy relies on comprehensive reports from Sprout Social to gauge user interaction and inform his content strategy. He emphasized the dual nature of analyzing social data–both quantitative, relying on the Sprout reports, and qualitative, understanding the fluid nature of social trends. Tommy is already utilizing generative AI for content creation, which sparked the idea of enhancing the Sprout Social reports’ analysis with a tool like Maizey.
With this knowledge and Tommy’s openness to integrating new tech, I began to upload Sprout Social reports to Maizey, training it to generate actionable insights from our social media data. This involved prompts that could lead Maizey to draw meaningful conclusions by comparing metrics across different social platforms, dates, times, and content types.
The process of creating a Maizey was daunting. But, after beginning, I was reaffirmed of the tool’s accessibility despite its sweeping technical capabilities. Joining an MCommunity group, titling the project, and uploading the relevant datasets were straightforward. Yet, I encountered an issue when I realized Maizey prefers text formats over processing structured data such as tables or images. Following recommendations from the GenAI team, I opted to convert the Sprout report PDFs into text documents–a temporary setback but a vital factor in ensuring correct responses.
With the upload complete, I began to explore the scope of this Maizey. The chatbot aided in efficiently pulling metrics and comparing platform engagement stats. Also, pointing out trends and differences between content types and what posts best appeal to different platforms. However, the further I dialed into specifics–such as understanding the impact of particular hashtags–the more I realized Maizey’s advice came with a caveat: a person is needed for depth and context.
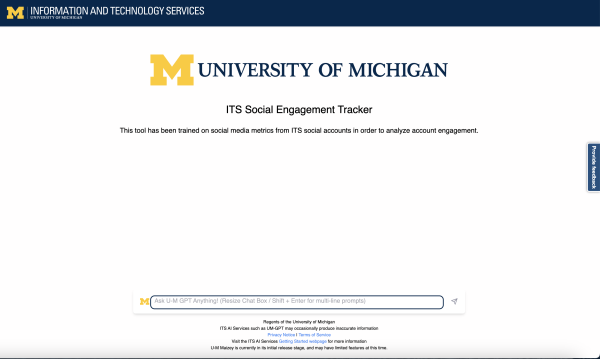
This ongoing development of AI analysis alongside human interpretation highlighted a central theme: this Maizey will never replace the need for Tommy, but is a helpful supplement to enhance efficiency. As I continue to work with Tommy, he’s helped to identify areas where these insights could be applied to our current social media strategy.
Over the next few weeks, I aim to refine Maizey’s training, coordinating with the GenAI team as they improve its capabilities with structured data and diverse input sources. Ultimately, Maizey’s application relies on partnership, where AI can aid in efficiency, and human experience provides perception, ensuring we stay connected and relevant in the dynamic world of social media.
I was able to:
- Quickly analyze engagement across platforms.
- Gain insights on how to increase engagement per platform.
- Create a posting schedule based on engagement analysis.
- Determine posting/content that is tailored to the differences between the platforms.
I ran into limitations with:
- Having to parse engagement data from PDF to unstructured document form.
- Not being able to upload data from different sources (i.e., engagement metrics are in doc format, and social media sites are links).
- Possibilities of hallucinations that require double checking of information.
- Limited knowledge of the social media platforms.
- Engagement metrics are not dynamic documents; therefore, with each new report, someone must manually reindex data.