Last time, I created a Maizey that helps researchers with menial tasks like summarizing literature reviews, identifying methods, and highlighting key findings. It works, but let’s face it, I’m no subject-matter expert. My one semester of experience in a college lab is measly compared to many of my research savant peers. In an effort to identify areas of improvement, I sought out my friend Lillian Shern, who has 48 months of research experience under her belt.
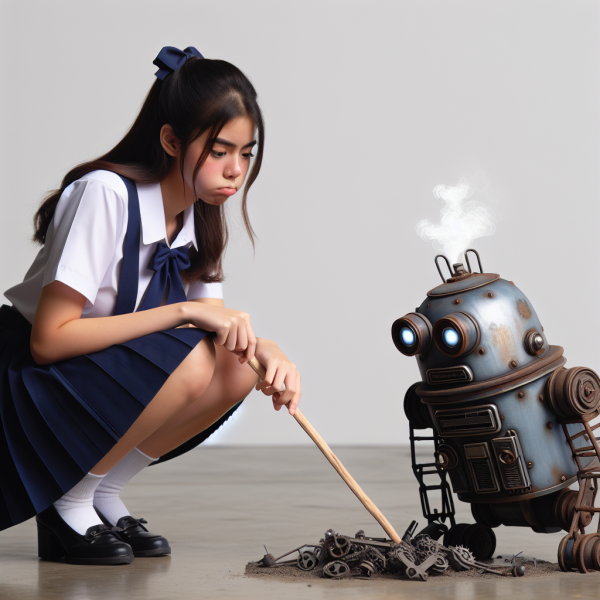
Shern’s research experience primarily involves qualitative research, including extensive literature reviews, data organization, and survey creation. Shern has seen both sides of the coin: research pre- and post-AI. Before the incorporation of AI, she would manually gather research papers from university libraries and Google Scholar, then organize them in Excel by identifying key variables such as dependent, independent, mediating, and moderating variables. This process, while thorough, is time-consuming and labor-intensive, she noted.
In her current workflow, Shern often utilized ChatGPT. She would input the abstracts of research papers into ChatGPT and ask it to identify the key variables from the abstract. This approach helped speed up the process of synthesizing information, though it had its limitations. Hallucinations occurred, and sometimes the abstract was too abstract: it did not contain enough information, especially for mediating and moderating variables.
When Shern tried out Maizey without any prior knowledge of how it works, she immediately gravitated toward her usual method: paste in the abstract and ask it to identify independent, dependent, moderating, and mediating variables. This was fascinating to observe and it was exciting to see experience at work. I will be shamelessly copying her method: I will modify the system prompt to let Maizey focus on the abstract instead of the entire paper, which can be dependent on graphics that are difficult for AI to index — at the time of writing, as this technology will outpace us all.
Shern perceived Maizey’s responses to be slower than ChatGPT’s as it outputs the response only when it’s done as opposed to streaming the response in real time. On the flipside, she noted that Maizey offered the advantage of directly citing sources from Google Drive, making citations easier. She also observed that Maizey’s identification of variables still involves some hallucination, often producing “variables that are not there,” but appreciated its ability to suggest possible moderating and mediating variables, even when they were not explicitly mentioned in the abstracts.
For Shern, the biggest edge that Maizey has over ChatGPT is its security aspect. Maizey doesn’t utilize any user input to train its model and no one at the University — not professors, not even ITS — can ever request to view the data. As she is taught by her Principal Investigator to not put sensitive research data into ChatGPT, she acknowledged that Maizey’s secure handling of data could make it a preferable choice for more sensitive research tasks beyond literature review.
Shern’s experience underscores the promising capabilities of Maizey as a research assistant. At the moment, her lab’s usage of generative AI is limited to preliminary tasks and much of the interpretation is still reserved for human researchers.
“We can use generative AI in terms of prompting them with the existing information,” Shern said, “Anything of predictive nature … could go really weird so you don’t want that.”
While there are areas for improvement, the AI’s ability to streamline literature reviews and provide secure, properly-sourced responses can support students navigating the oceans of text. As Maizey continues to develop, it holds the potential to transform the research experience at the University, making it more efficient and accessible for students like Shern.