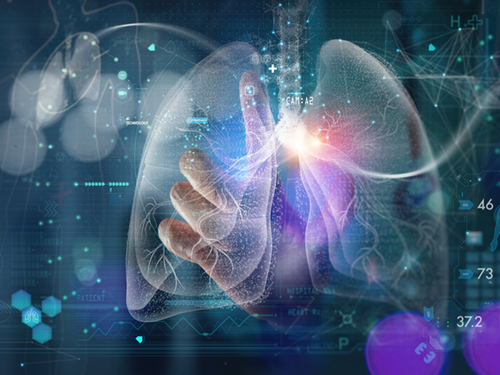
Acute respiratory distress syndrome (ARDS) is a deadly critical illness that has a high mortality. However, recognition and diagnosis of ARDS is often missed or delayed, and patients do not receive evidenced-based care when ARDS goes unrecognized.
To help physicians identify ARDS faster and more reliably, researchers at U-M’s Max Harry Weil Institute for Critical Care Research and Innovation and Michigan Medicine developed a deep learning algorithm trained to detect ARDS findings in chest X-rays. Now, in a new study published in npj Digital Medicine, the team examined the unique strengths and weaknesses of this model compared to those of human expert physicians. They also explored how a model and physicians could potentially work together to improve ARDS diagnosis, ultimately improving outcomes for patients.
“Thanks to recent advances in artificial intelligence (AI), we have deep learning systems that can diagnose health conditions based on clinical images with expert-level accuracy,” said Dr. Negar Farzaneh, a Weil Institute Research Investigator and Data Scientist, as well as lead author on the study. “But we’re also seeing a gap between studies describing the capabilities of these systems and efforts to investigate how or when to integrate them in a manner that supports physicians and improves diagnosis. That gap is something we wanted to address in our study.”
Using a reference standard of 414 chest X-rays from adult hospital patients with acute hypoxic respiratory failure, the team deployed the AI model alongside a group of physicians who had expertise in chest x-ray interpretation for ARDS detection. To determine the strengths and weaknesses of both groups, the team measured three factors: overall performance in ARDS detection, accuracy based on difficulty of X-ray interpretation, and level of AI/physician certainty in their interpretations.
Compared to the physicians, the AI model demonstrated higher overall performance in detecting whether ARDS findings were present. But while the model had a stronger showing at first, the team hypothesized that X-ray difficulty may play a key role.
To explore this concept further, the team divided the X-rays based on how challenging they were to classify–with “difficult” defined as those in which there was disagreement among the physicians regarding the interpretations. The researchers found that the AI model outperformed the physicians in interpreting chest X-rays that were not as difficult to review. However, the physicians were better at reviewing the minority of chest X-rays that were more difficult to review. Both physicians and the model also rated their confidences in the chest X-ray interpretation, and the team found that when one was less confident the other performed better.
“It’s interesting to see how the AI model and physicians can complement each other’s strengths. In situations where physicians lacked confidence in interpreting a chest X-ray, the AI model provided more accurate results, and vice versa,” said Dr. Farzaneh.