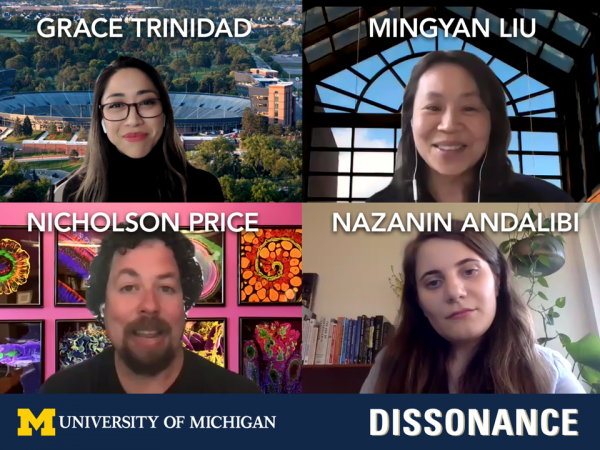
Artificial intelligence, machine learning, and algorithmic decision making are not science fiction. Machines are making decisions for us, whether in connected cars or in hiring, home loans, policing, and more. It’s important to engage with diverse people to account for our human values and perspectives when developing algorithms and other software, according to a panel of U-M experts who discussed the film Coded Bias at a Dissonance Event on April 15.
ITS Information Assurance, the School of Information, and the Law School’s Privacy, Technology and Law Association sponsored free online access to the film for a week, and then the Dissonance organizing committee brought the panelists together for an online discussion (watch a recording of the panel discussion).
Discovering racial and gender bias
Coded Bias follows the journey of Joy Buolamwini, a computer scientist and digital activist based at the MIT Media Lab, as she worked with others to push for the first legislation in the U.S. to govern against bias in artificial intelligence (AI) algorithms. Buolamwini began her journey with the discovery of racial and gender bias in AI algorithms used in facial recognition software, noting that people of color and women are vastly underrepresented in the databases that feed the algorithms.
“We should not assume that inclusion in data sets is necessarily desired,” noted Nazanin Andalibi, an assistant professor of information in the U-M School of Information and an assistant professor at the Digital Studies Institute in the College of Literature, Science, and the Arts (LSA). She said, “We should sometimes ask if the technology needs to exist at all.” She pointed out that while more diverse data sets can reduce bias, “it is important to respect and support people’s agency and realize that not all bias will be removed through technical fixes.”
Don’t try to replace humans
“I like the idea of intelligent assistance (IA) rather than artificial intelligence (AI),” said Mingyan Liu, the Peter and Evelyn Fuss chair of electrical and computer engineering in the U-M Electrical Engineering and Computer Science (EECS) Department. “I don’t see why the goal has to be to replace humans; why is the goal not to make humans better humans?”
She questioned the faith people seem to have in algorithms: “At the end of the day it is a piece of software. We’ve all seen sloppy software. Why do we think of AI differently?”
Liu quoted from the movie, that machine learning is about using historical information to make predictions about the future, and there are limits to what AI can do. It may be good at monitoring thousands of patients, for example, but, she said, “it is not good at sitting down and talking with someone about their family history.”
Moderator Grace Trinidad, an Ethical, Legal, and Social Implications (ELSI) postdoctoral fellow in the U-M School of Public Health, followed up, asking, “What is intelligence in this context? What is it that we should be in pursuit of?”
“Algorithms are very good at rule-based activities,” said Liu. “They are good as an initial filter in terms of doing things at scale, but not as being the final arbiter.” She said there are many areas that machines are just not very good at: “Things like understanding emotions, reading between the lines, hearing the unspoken word. Things we do so naturally as humans can be incredibly challenging for machines.”
Transformative opportunities
“I’m still really excited about the things AI can do, hopefully, to transform health care,” said Nicholson Price, professor of law at the U-M Law School. “We can make specialized care available more broadly.” He added, “Lots of exciting things are being developed at the University of Michigan and other universities.”
Price stressed the need to collect data not just from major medical centers but from small hospitals and rural health clinics for more representative algorithms. “The dream of democratizing expertise isn’t realized if the data is all from similar places.”
“Technology alone is not going to solve this problem,” said Liu. “The ultimate solution is going to use one part regulation and policy and one part social and community support.”
Trinidad asked the panelists how AI might be regulated to reduce bias.
Transparency needed for regulation
The panelists called out the need for meaningful algorithmic transparency. “The secrecy makes it incredibly difficult to know when there is bias going on, makes it hard to regulate,” said Price. Greater transparency could enable better oversight of company algorithms by government, academics, and activists.
Andalibi added that she is excited about the potential for collective action and different forms of resistance to make companies take greater responsibility about the impacts, including downstream impacts, of their products.
Regulators are already exploring possibilities. On April 21, European Union officials outlined proposed regulations for AI.
Access to Coded Bias and the panel discussion were sponsored by the Dissonance Event Series, ITS Information Assurance, the U-M School of Information, and the U-M Law School’s Privacy and Technology Law Association.
The Dissonance Event Series engages in multi-disciplinary conversations at the confluence of technology, policy, privacy, security, and law.