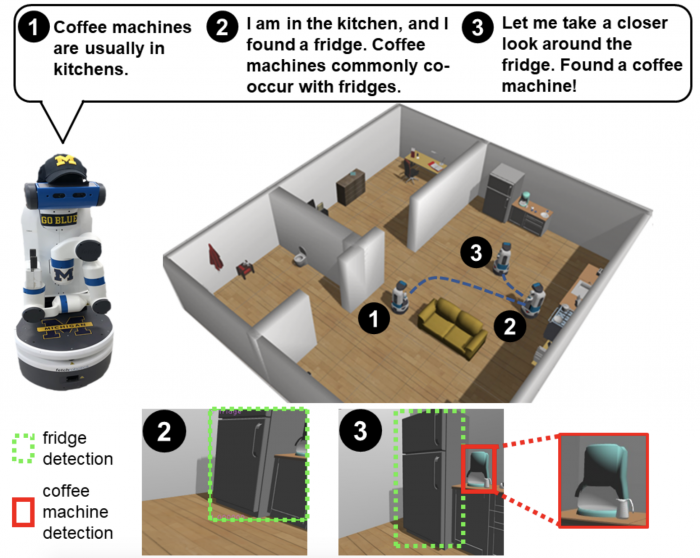
Robots can learn how to find things faster by learning how different objects around the house are related, according to work from the University of Michigan. A new model provides robots with a visual search strategy that can teach them to look for a coffee pot nearby if they’re already in sight of a refrigerator, in one of the paper’s examples.
The work, led by Prof. Chad Jenkins and CSE PhD student Zhen Zeng, was recognized at the 2020 International Conference on Robotics and Automation with a Best Paper Award in Cognitive Robotics.
A common aim of roboticists is to give machines the ability to navigate in realistic settings – for example, the disordered, imperfect households we spend our days in. These settings can be chaotic, with no two exactly the same, and robots in search of specific objects they’ve never seen before will need to pick them out of the noise.