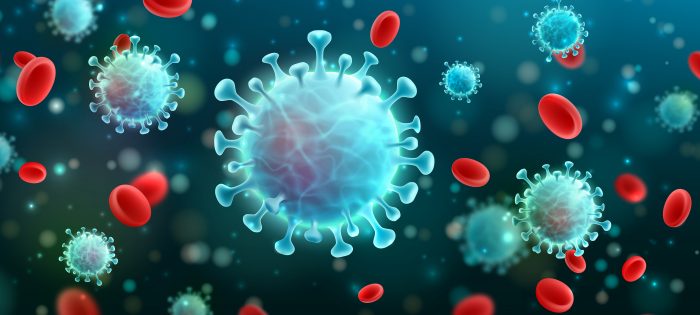
Amid a growing coronavirus crisis, experts in all fields have begun compiling massive datasets to track the impact of the contagion. To make constructing these datasets as accurate and timely as possible, Michael Cafarella, professor of computer science and engineering, is leading an NSF-funded project that will build high-quality auxiliary datasets to enable automatic quality checking and fraud detection of the new data.
Rapid analytical efforts by policymakers, scientists, and journalists rely on coronavirus data being complete and accurate. But like all dataset construction projects, those chronicling the coronavirus are prone to shortcomings that limit their effectiveness if left unaddressed. These issues include messy or unusable data, fraudulent data, and data that lacks necessary context. Automatically checking coronavirus datasets against the pertinent, related datasets provided by Cafarella’s team can make them more effective and insightful.