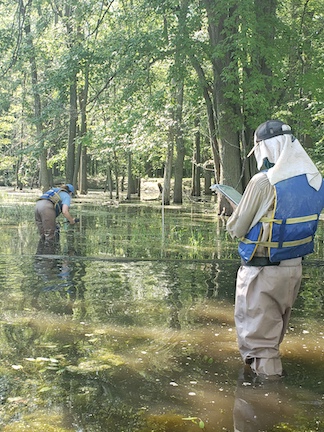
A U-M School for Environment and Sustainability (SEAS) student team is working with the Shiawassee National Wildlife Refuge to study how fish move through different wetland habitats. Their work is primarily dependent on being in the field, but in March the pandemic delayed fieldwork. In June, the team of SEAS master students was allowed to begin socially distant field work. But the question was: How?
With the help of the ARC-TS Scientific Computing and Research Consulting Services, the SEAS students were able to pivot their research methodology and develop advanced analysis approaches for hydroacoustic data using strategically placed cameras and machine learning.
The Shiawassee refuge is divided into separately managed wetland units. These wetland units can be connected or cut off from one another and the Shiawassee river. An Adaptive Resolution Imaging Sonar (ARIS) camera has been placed at the connection point between the refuge’s “control” wetland units and the river to track fish movements between these two ecosystems. They are created through human-made dikes and water control structures.
In order to find answers about fish movement, the SEAS team is divided into three separate parts:
- In-the-field monitoring of fish, macroinvertebrates, water quality, and vegetation
- ARIS camera work: understanding how to use ARIS footage to answer ecological questions using machine learning facilitated by the ARC-TS Data Consultation Service
- Community education and outreach regarding restoration work at the refuge
Meghan Richey, machine learning specialist, and Armand Burks, research data scientist, are part of the ARC-TS Data Science Consultation team. Together they are working to see the project through by understanding the needs of the SEAS research team and providing the necessary coding expertise. In addition, they are working to provide the SEAS team with tools to become independent programmers so they can implement programming/coding into their future research endeavors.
Richey works with the machine learning team. Machine learning is a tool for turning information into knowledge. It automatically finds patterns in complex data that are difficult for a human to find. While traditional problem solving uses data and rules to find an answer, machine learning uses data and answers to find the rules that apply to a problem. Together, they count the number of fish moving in front of the camera that was originally placed in mid-March but removed in mid-May due to flooding from the dam breaches in Midland, Mich. The camera has since been placed back into the “avenue” between one of the managed wetland pools and the river. With the help of a written machine algorithm, Richey and the SEAS team are able to count the number of fish they’re seeing in front of the camera feed. There is one camera placed in the water that is taking underwater images of the fish. The fish swim by the camera, and the team captures these frames.
Burks is responsible for the data conversion stages of the project. “They have a large amount of data that’s generated from the underwater camera. These aren’t the typical cameras as we would think of; they work with sonar which is based on sound. It is generating a lot of data in this sound-based sonar format that needs to be converted into something that is usable by the machine learning model.”
In order for the program to run smoothly and be able to count the fish, Burks and SEAS team had to develop a tool that allows them to turn the raw data into an actual video feed. Once this is completed the SEAS research team watch a series of pre-recorded videos that are saved to files. In order to receive the raw data, a large data conversion must happen to transform raw sonar data into videos. From there, the machine learning algorithms can be built and analyzed.
The ARC-TS team plans to continue working with the students and the team at the refuge to refine their methods and test with recently collected footage.
ARC-TS is a division of Information and Technology Services (ITS).