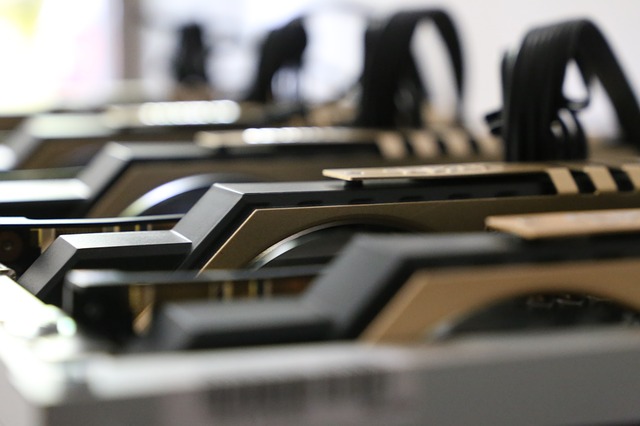
Graphics Processing Units (GPUs) have been getting a big workout from new advancements in AI because they offer significant performance boosts thanks to their parallel computing capabilities. GPUs are employed by large computing clusters to handle huge datasets for deep learning applications. Achieving cost-effectiveness in these clusters relies on efficiently sharing resources between multiple users.
However, these systems are not designed for efficient, fine-grained sharing of GPU resources at either the micro or macro scale. Memory and computation power are regularly wasted at the level of individual GPUs and at that of an entire GPU cluster.
Professor Mosharaf Chowdhury is working to overcome both of these shortcomings, multiplying the number of jobs a cluster can finish in a set amount of time and streamlining methods of sharing resources on the fly. His team has described a set of solutions to achieve efficient GPU resource sharing at multiple scales: both within a single GPU and across many GPUs in a cluster.